Unveiling DALM: Revolutionizing Enterprises with Domain Adapted Language Model Systems
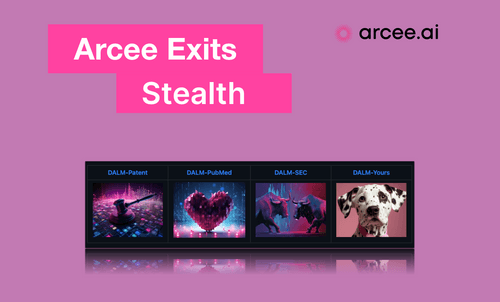
In the rapidly evolving landscape of Generative AI, the quest for more refined and contextually aware language models has led to our development of the Domain Adapted Language Model System (DALM). This innovation promises to reshape how enterprises harness the power of language models tailored to their specific domains. In this blog, we dive into the specifics of DALM, its three-fold approach, and the benefits it brings to anyone wanting more domain focused models.
The Foundation of DALM: Pretrained In-Domain Large Language Models
Large Language Models (LLMs) represent a type of artificial intelligence that undergoes training on an extensive amount of web data and various online resources. This training enables them to produce responses to language-based inquiries that closely resemble human expression. Despite the impressive achievements already demonstrated by commercial and versatile LLMs such as ChatGPT, BARD, and Claude, the prospective evolution of LLMs is trending towards models that are more focused on particular domains, possessing compactness and enhanced efficiency. Enterprises are striving to tailor these models to specific requirements, thereby removing the necessity for the expansive and all-encompassing data presently employed by their large foundational models.
Introducing DALM: a foundational strategy built upon the utilization of pretrained, in-domain Large Language Models. Initially, these in-domain LLM’s encountered dual challenges—limited access to openly available checkpoints and the presence of exceedingly large models like the 1.7 trillion parameters of GPT-4. However, the landscape of LLM research has evolved. There's a notable shift towards fully open-sourcing LLM checkpoints, featuring commercially viable sizes ranging from 3 to 13 billion parameters. These LLMs have undergone pre-training with trillions of tokens. This evolution strikes a great balance between size and functionality, rendering them exceptionally suitable for tasks demanding specialized or niche language comprehension. Within the framework of DALM, our primary focus lies in constructing systems by customizing publicly available LLM checkpoints with domain-specific data. To achieve this objective, DALM emphasizes the utilization of innovative Parameter Efficient Fine Tuning (PEFT) techniques like LORA and Quantization, which strike a balance between performance and computational expenditure.
As these in-domain LLMs are the core of DALM, they bring in a new wave of precision and adaptability. Arcee is actively developing in-domain models tailored for patent lawyers, biomedical, SEC filings, and the insurance sector, among other specific domains. Enterprises, regardless of their domains, can now unlock the true potential of large language models, seamlessly integrating them into their operations to drive insights, innovation, and informed decision-making. Through the deployment of in-domain LLMs into their own architecture, DALM signifies Arcee’s bet of a world of millions - if not billions of smaller, in-domain models, over the one-model-rules all AGI world.
Subsequently, we discuss each stage of the DALM techniques.
Context-Based Generator Alignment
Within DALM, we now take the approach of in-context fine-tuning for pre-trained LLMs using domain-specific datasets. This strategic step capitalizes on the inherent potential of the pre-existing model, thereby refining its contextual comprehension in alignment with the specific requirements of many organizations.
Furthermore, we acknowledge the potential challenge of data scarcity, particularly concerning in-domain instruction datasets. In response, we dedicate attention to innovating novel techniques for generating datasets that facilitate the domain adaptation process. As highlighted earlier, we deploy methodologies such as QLORA, which ingeniously amalgamate the principles of PEFT and model quantization, resulting in computational resource-friendly procedures.
The Essence of Domain Adaptation: Refining with Retrieval
Retrieval Augmented Generation (RAG) plays an important role in domain-specific applications, as it enables domain-adapted LLMs to transcend their parametric memory, engaging with custom and evolving knowledge bases. Within this context, we have identified the retriever as a key component in the RAG pipeline and have concentrated on effecting domain adaptation of the retriever during our second phase. Through orchestration, the retriever model is subjected to training utilizing in-domain contextual data. This strategic maneuver empowers the system to retrieve domain-specific information, thereby establishing a solid foundation for seamless context integration. Much like the context instruction fine-tuning phase, we have also devised strategies to contend with data scarcity and the computational overhead.
In this phase, DALM effectively bridges the gap between the inherent capabilities of the LLM and the precise requisites of a companies domain. By endowing the retriever model with the ability to comprehend the subtleties that characterize a specific vertical, DALM ensures that the domain retrieval process is not only efficient but also accurate compared to other generic vector stores.
Bringing it all Together: End-to-End Contextual Awareness
While the current architecture of RAG emphasizes the separation between a retriever and a large language model (LLM), the original concept of RAG aimed to synergize retrieval and generation. To enhance clarity, we now refer to this as "RAG-end2end." Within the framework of RAG-end2end, our primary objective is to train a retriever and a generator in a fully differentiable manner.
Although RAG-end2end is not a new idea, prior efforts mainly involved coupling a dense retriever with a sequence-to-sequence generator like BART or T5. It's important to note that implementing RAG-end2end within the context of an LLM can be computationally intensive. At Arcee, we are dedicated to exploring RAG-end2end solutions that address the aforementioned challenges. This is particularly compelling as there is existing evidence suggesting that RAG-end2end has the potential to enhance both retrievers and generators while mitigating issues such as generator hallucinations.
In this context, we have introduced an innovative RAG-end2end pipeline. Our approach draws inspiration from the concept of in-batch negative learning, a prominent technique in contrastive learning. Not only does our method efficiently facilitate the end-to-end process, but it also achieves a groundbreaking milestone by seamlessly integrating a retriever with a generative language model within an end-to-end framework.
The Power of DALM
The convergence of these three phases in an end2end framework results in the birth of a fully Domain Adapted Language Model system, poised to revolutionize how organizations build LLM stacks. The benefits are manifold: increased efficiency, accuracy, and contextual awareness. By seamlessly integrating DALM into their operations, businesses gain a competitive edge, making more informed decisions and deriving insights that are laser-focused on their domain.
Stay tuned for our DALM paper, which will be an in-depth technical overview and comprehensive benchmarking analysis of our RAG-end2end framework, shedding light on DALM’s advanced capabilities and performance metrics.