March is Merge Madness
To celebrate Arcee’s recent merger with mergekit, we’re bringing you a month of resources and knowledge on model merging.
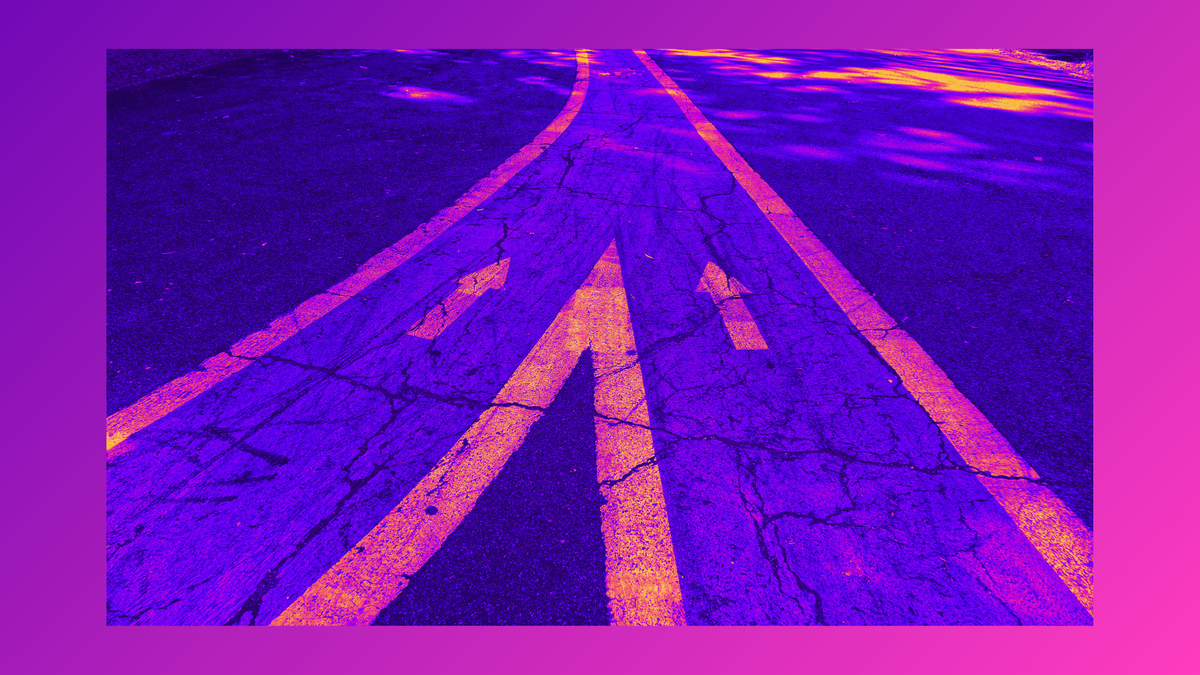
It will be everything you've wanted to know about model merging, and more. Over the next four weeks, we’ll be bringing you tips and tutorials on:
- How model merging works
- The massive $$$ savings of model merging
- Industry verticals where model merging is particularly useful
- Why Arcee and mergekit joined forces.
Have questions about model merging? Send them to our team on X or LinkedIn. And check out this interview with our CEO Mark McQuade who explains why Arcee is committed to becoming the leader in model merging, and how model merging fits into Arcee’s Small Language Model (SLM) system.
Arcee CEO Mark McQuade on where model merging fits in the SLM system.
TRANSCRIPT:
Mark McQuade, Arcee CEO
We created a larger system called an SLM adaptation system, and it has multiple layers of domain adaptation, one being Continual Pre-Training, then Alignment, which is Supervised Fine-Tuning, and then finally Retrieval Augmented Generation.
It's kind of those three pillars that are our SLM Adaptation System.
So where model merging and mergekit fit into our system is in the very first layer in Continual Pre-training – while you have models that you can train,smaller models that you can train on a much more efficient pace and then you can merge them with larger models, right? So in the world of Continual Pre-Training that exists today, you have to train over the entire model, right? Now… we're saying – don't trainover the entire model, train a much much smaller model and then merge it with a much larger model or, you know, SLM kind of stands for small… So we don't actually believe in that large of a model… We think that 99% of business use cases can be solved with smaller models.
So it's just a matter of training that smaller model and merging it with a larger model to allow for the flexibility to have, you know, a great performing model that you did not need to train over every parameter & you didn't needto spend hundreds of thousands to do it. So it's a perfect fit.