7 Billion Reasons to Choose Arcee-Meraj-Mini: The Open-Source Arabic SLM for All
Hot on the heels of our top-performing 72B Arabic-language model Arcee-Meraj, we bring you a 7B version: Arcee-Mini-Meraj, which boasts exceptional performance in instruction-following, generating long texts, structured data understanding, and generating structured outputs.
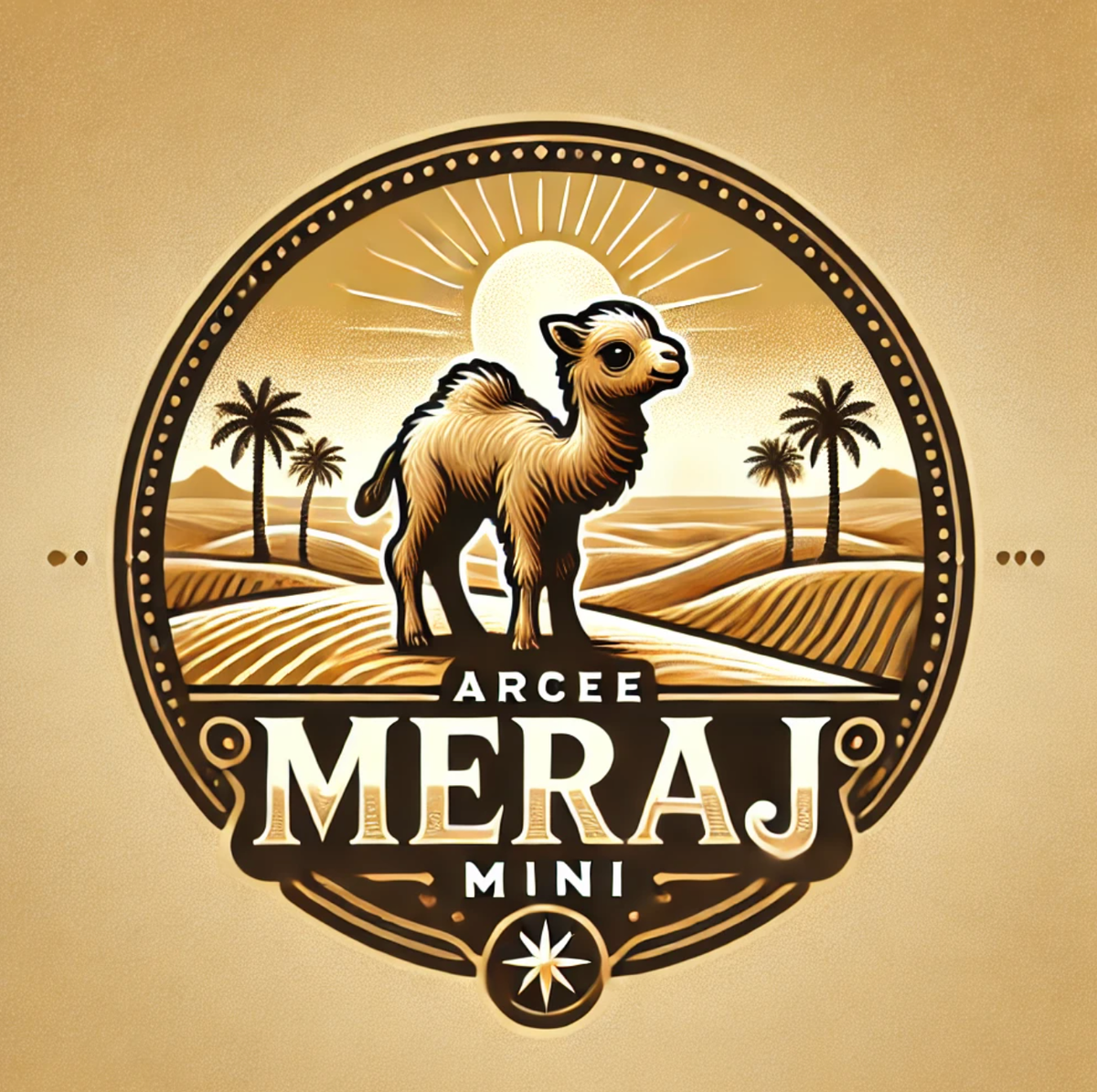
Following the release of our chart-topping 72B Arabic-language model Arcee-Meraj, trained on Qwen2-72B-Instruct, we're thrilled to unveil a more compact version: Arcee-Meraj(معراج)-Mini.
This open-source small language model (SLM), meticulously fine-tuned from Qwen2.5-7B-Instruct, is crafted specifically for the Arabic language.
Qwen2.5 is the latest version of Qwen series of models as of October 2024. It has been trained on a very large dataset, totaling up to 18 trillion tokens, and offers enhanced capabilities and performance, compared to its previous version Qwen2, particularly in coding and mathematics. Qwen2.5 improves on:
• instruction-following
• generating long texts (over 8K tokens)
• structured data understanding (i.e., tables)
• and generating structured outputs (especially JSON).
Qwen2.5 supports an impressive array of 29 languages, including Arabic, making it an effective multilingual model for diverse linguistic tasks. Its extensive language coverage is a crucial factor in integrating it, as it enables effective adaptation to a wide range of linguistic needs.
Technical Details
Arcee-Meraj-Mini is a 7B parameter model designed to excel in both Arabic and English and to be easily adaptable to different domains and use cases.
Below is an overview of the key stages of the development of the model:
- Data Preparation: We filtered candidate samples from diverse English and Arabic sources to ensure high-quality data. Some of the selected English datasets were translated into Arabic to increase the quantity of Arabic samples and improve the model’s quality in bilingual performance. Then, new Direct Preference Optimization (DPO) datasets were continuously prepared, filtered, and translated to maintain a fresh and diverse dataset that would support better generalization across domains.
- Initial Training: We trained the Qwen2.5 model with 7 billion parameters using these high-quality datasets in both languages. This allows the model to handle diverse linguistic patterns from over 500 million tokens, ensuring strong performance for both Arabic and English tasks.
- Iterative Training and Post-Training: Iterative training and post-training iterations refined the model, enhancing its accuracy and adaptability to ensure it would perform well across varied tasks and language contexts.
- Evaluation: Arcee-Meraj-Mini is based on training and evaluating fifteen different variants to explore optimal configurations – with assessments done on both Arabic and English benchmarks and leaderboards. This step ensures the model is robust in handling both general and domain-specific tasks.
- Final Model Creation: We selected the best-performing variant and used the MergeKit library to merge the configurations, resulting in the final Arcee-Meraj-Mini. This model is not only optimized for language understanding, but also serves as a starting point for domain adaptation in different areas.
With this process, Arcee-Meraj-Mini has been crafted to be more than just a general-purpose language model; it’s an adaptable tool, ready to be fine-tuned for specific industries and applications, empowering users to extend its capabilities for domain-specific tasks.
Performance Details
Open Arabic LLM (OALL) Benchmarks:
Arcee-Meraj-Mini consistently outperforms state-of-the-art models on most of the Open Arabic LLM Leaderboard (OALL) benchmarks, highlighting its improvements and effectiveness in the Arabic language, and securing the top-performing position on average among models of similar size.
Model Name | Average | ACVA | Alghafa | EXAMS | ARC Challenge | ARC Easy | BOOLQ |
---|---|---|---|---|---|---|---|
Arcee Meraj Mini | 0.56 | 0.48 | 0.62 | 0.52 | 0.66 | 0.70 | 0.72 |
Qwen2.5-7B-Instruct | 0.53 | 0.43 | 0.59 | 0.51 | 0.60 | 0.60 | 0.77 |
Arcee Spark | 0.53 | 0.49 | 0.60 | 0.48 | 0.56 | 0.63 | 0.78 |
Llama-3.1-8b-Instruct | 0.45 | 0.51 | 0.51 | 0.51 | 0.42 | 0.43 | 0.72 |
Aya-23-8B | 0.47 | 0.47 | 0.58 | 0.43 | 0.44 | 0.50 | 0.70 |
Jais-13b | 0.36 | 0.41 | 0.44 | 0.24 | 0.26 | 0.29 | 0.62 |
AceGPT-v2-8B-Chat | 0.50 | 0.50 | 0.60 | 0.52 | 0.52 | 0.59 | 0.62 |
Model Name | COPA | HELLASWAG | OPENBOOK QA | PIQA | RACE | SCIQ | TOXIGEN |
---|---|---|---|---|---|---|---|
Arcee Meraj Mini | 0.72 | 0.42 | 0.62 | 0.70 | 0.61 | 0.60 | 0.75 |
Qwen2.5-7B-Instruct | 0.69 | 0.43 | 0.54 | 0.71 | 0.55 | 0.57 | 0.79 |
Arcee Spark | 0.63 | 0.38 | 0.55 | 0.70 | 0.49 | 0.54 | 0.75 |
Llama-3.1-8b-Instruct | 0.58 | 0.27 | 0.44 | 0.62 | 0.43 | 0.59 | 0.43 |
Aya-23-8B | 0.46 | 0.32 | 0.49 | 0.64 | 0.41 | 0.51 | 0.44 |
Jais-13b | 0.49 | 0.25 | 0.32 | 0.54 | 0.22 | 0.31 | 0.47 |
AceGPT-v2-8B-Chat | 0.59 | 0.32 | 0.56 | 0.64 | 0.54 | 0.57 | 0.43 |
Translated MMLU:
We also focused on the multilingual (Arabic) MMLU dataset, as distributed through the LM Evaluation Harness repository, to benchmark state-of-the-art multilingual and Arabic models against Arcee-Meraj-Mini. Our results demonstrate that Arcee-Meraj-Mini consistently outperforms these competing models – showcasing its superior performance, robustness in handling diverse Arabic linguistic tasks, extensive world knowledge, and advanced problem-solving capabilities.
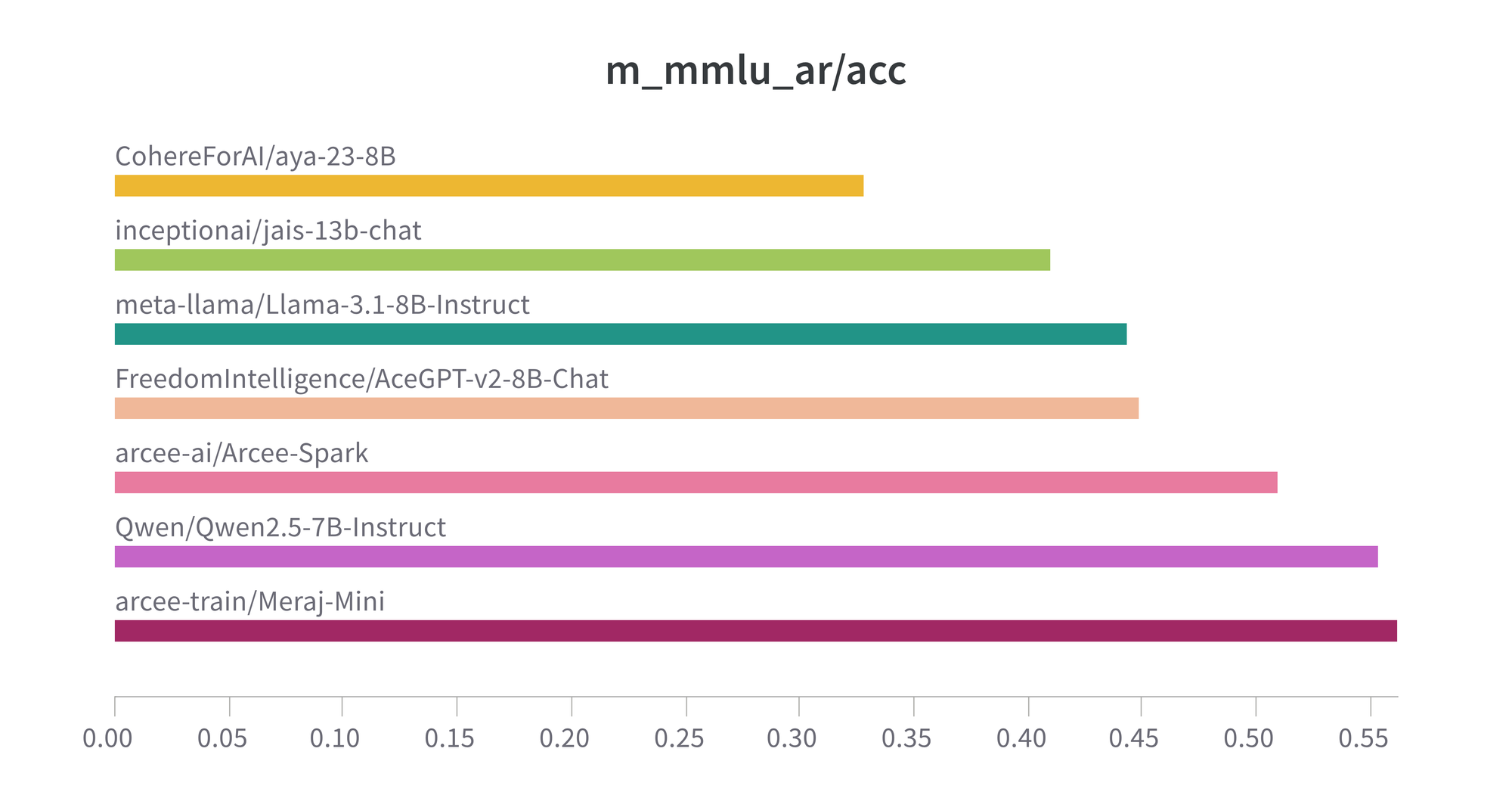
English Benchmarks:
Arcee-Meraj-Mini performs comparably to state-of-the-art models, demonstrating how the model retains its English language knowledge and capabilities while learning Arabic.
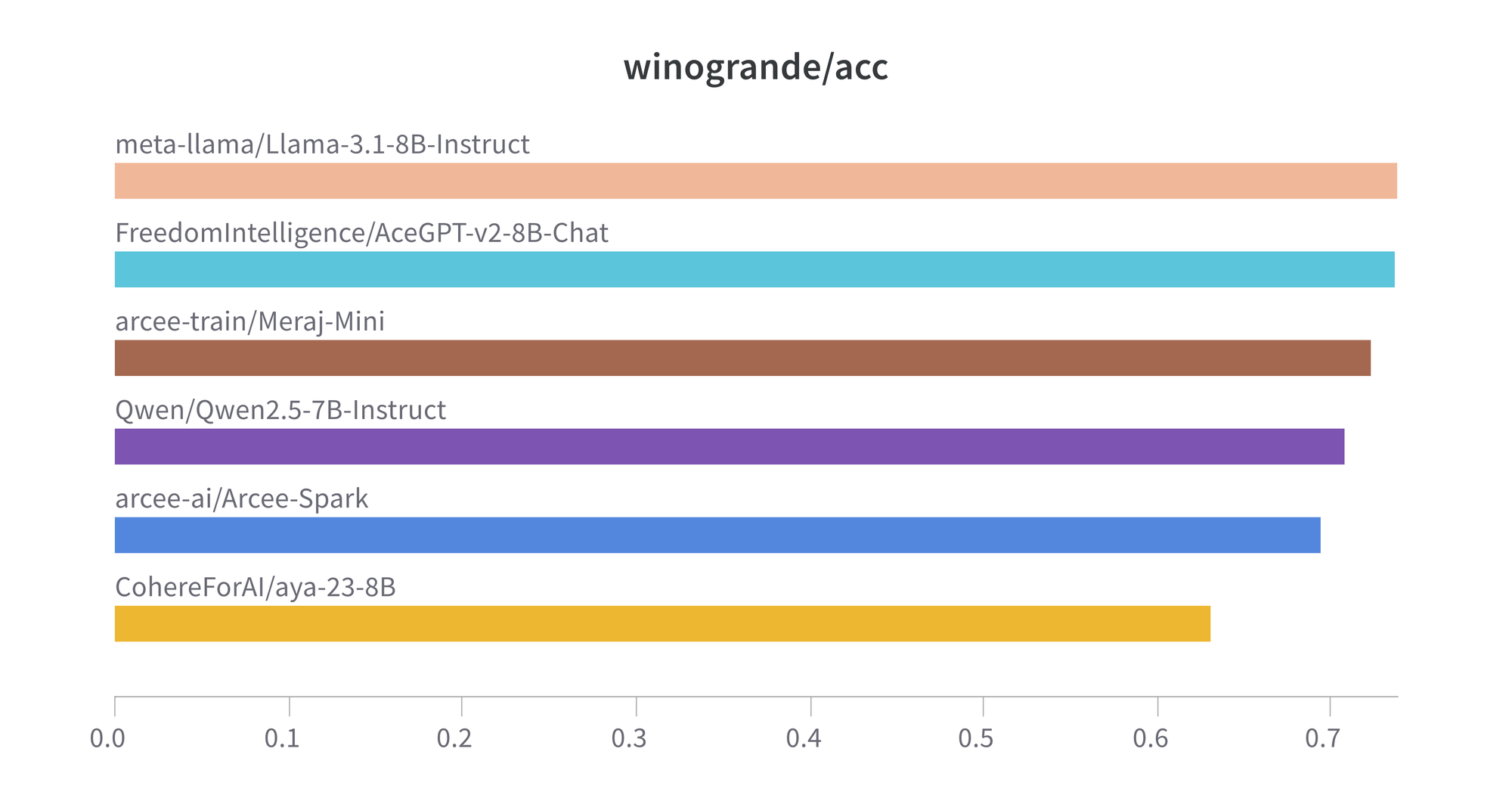
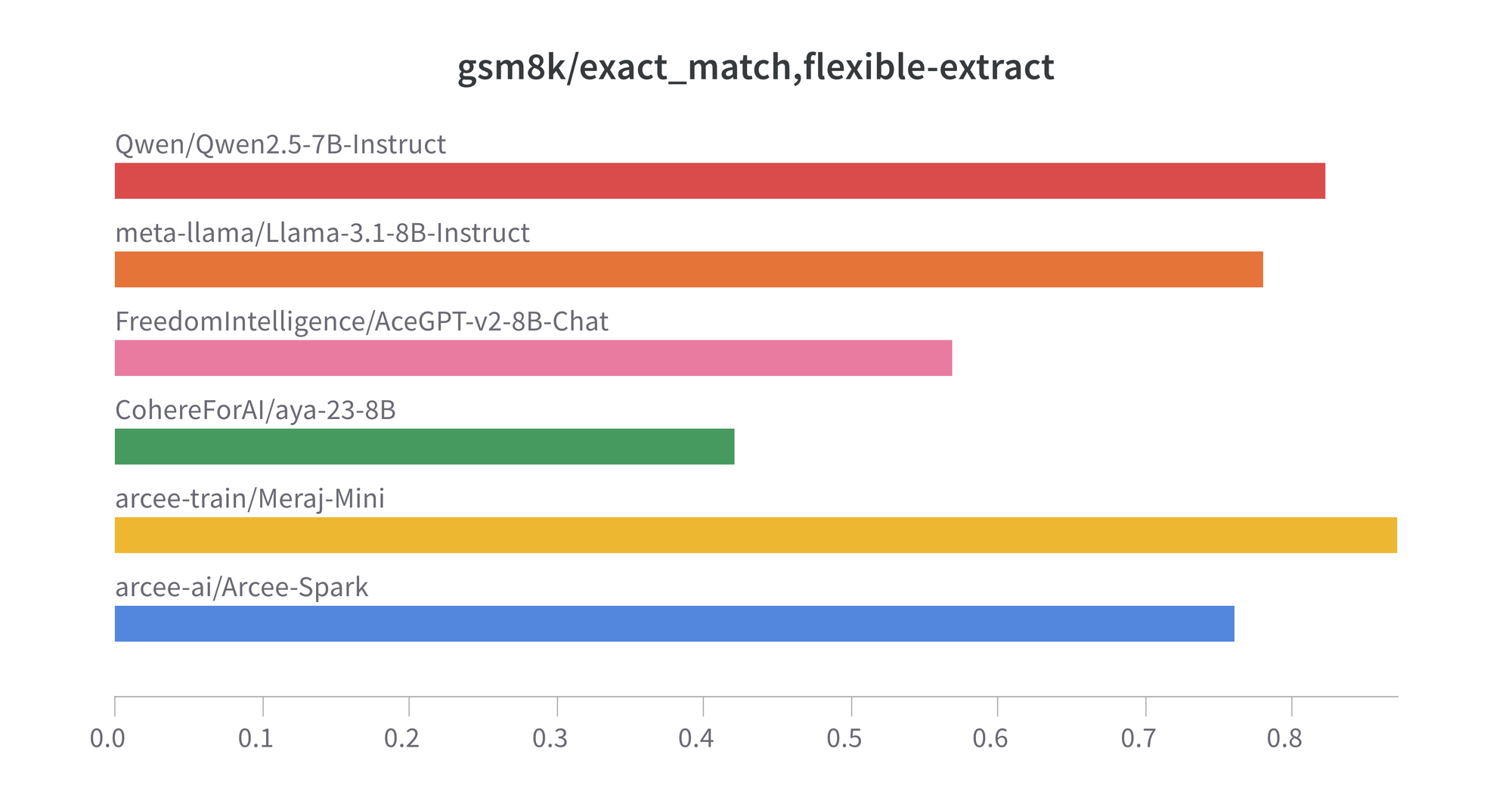
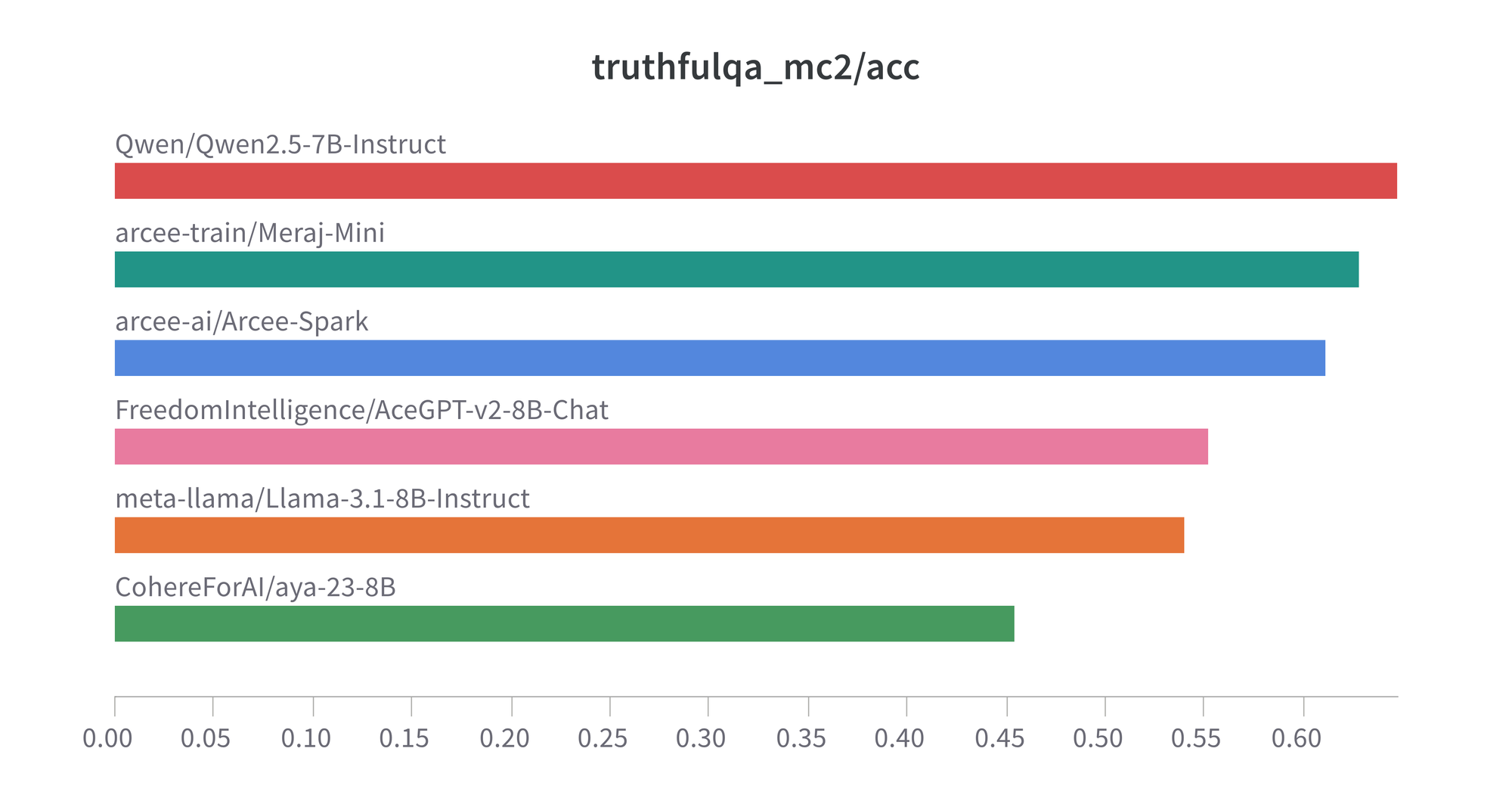
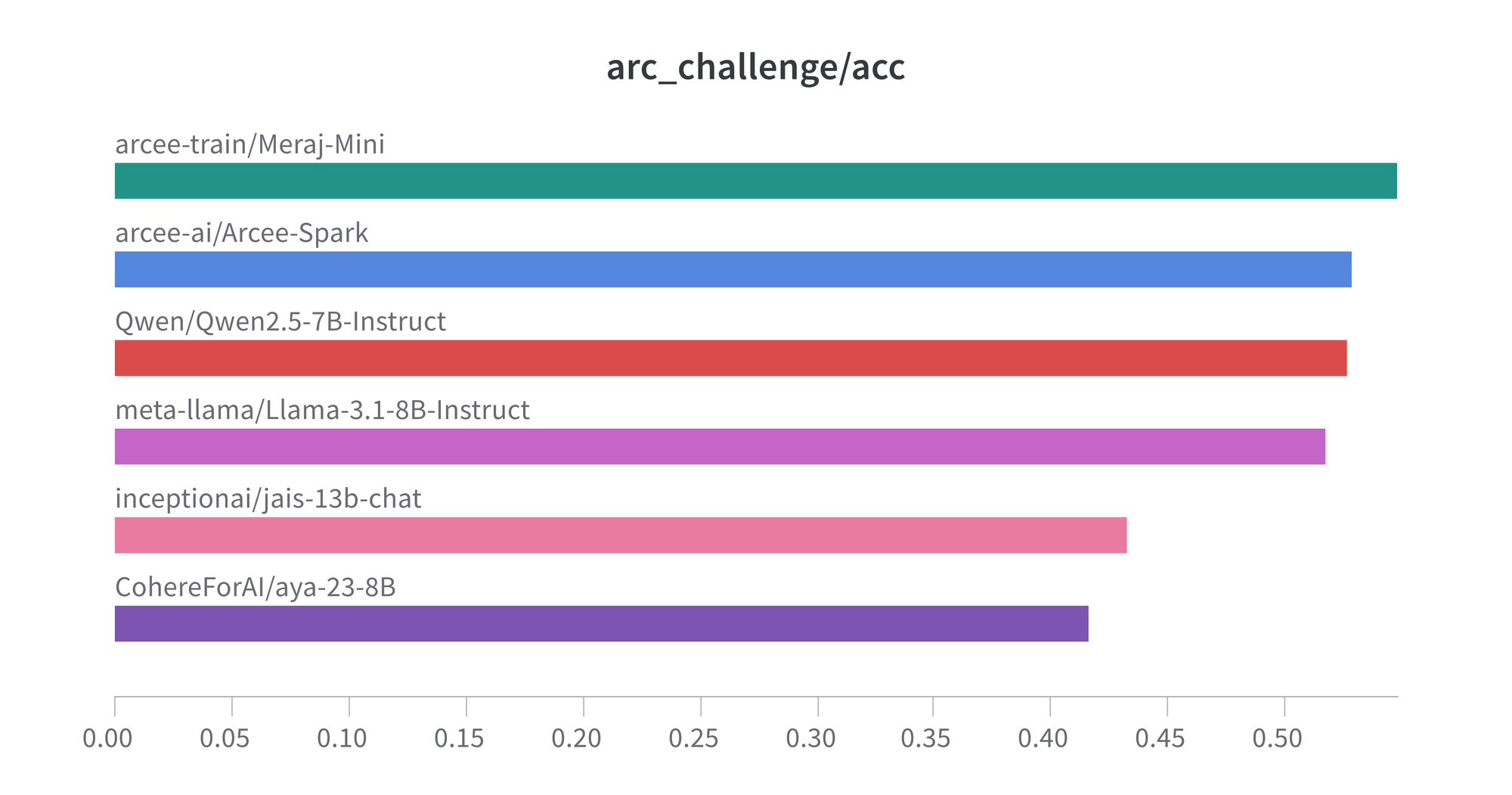
Key Capabilities
Arcee-Meraj-Mini is capable of a wide range of language tasks, including:
- Arabic Language Understanding: Arce-Meraj-Mini excels in general language comprehension, reading comprehension, and common-sense reasoning – all tailored to the Arabic language, providing strong performance in a variety of linguistic tasks.
- Cultural Adaptation: The model ensures content creation that goes beyond linguistic accuracy, incorporating cultural nuances to align with Arabic norms and values, making it suitable for culturally-relevant applications.
- Education: It enables personalized, adaptive learning experiences for Arabic speakers by generating high-quality educational content across diverse subjects, enhancing the overall learning journey.
- Mathematics and Coding: With robust support for mathematical reasoning and problem-solving, as well as code generation in Arabic, Arcee-Meraj-Mini serves as a valuable tool for developers and professionals in technical fields.
- Customer Service: The model facilitates the development of advanced Arabic-speaking chatbots and virtual assistants, capable of managing customer queries with a high degree of natural language understanding and precision.
- Content Creation: Arcee-Meraj-Mini generates high-quality Arabic content for various needs, from marketing materials and technical documentation to creative writing –ensuring impactful communication and engagement in the Arabic-speaking world.
Model Usage
You can find the detailed Model Card for the Arcee-Meraj-Mini model on Hugging Face here.
To try the model directly, you can try it out using this Google Colab notebook.
Bridging the Language Gap: Why Arabic Language Models are Essential for an Inclusive AI Future
Bringing support for the Arabic language to large language models (LLMs) is crucial for many reasons, ranging from promoting inclusivity to addressing unique linguistic and cultural characteristics.
Inclusivity and Accessibility
Arabic is spoken by more than 400 million people across more than 20 countries, yet it remains underrepresented in AI. Arabic-language LLMs make technology accessible to more people, and providing Arabic language support ensures that Arabic speakers can access AI tools in their native language – breaking down language barriers and making technology more inclusive.
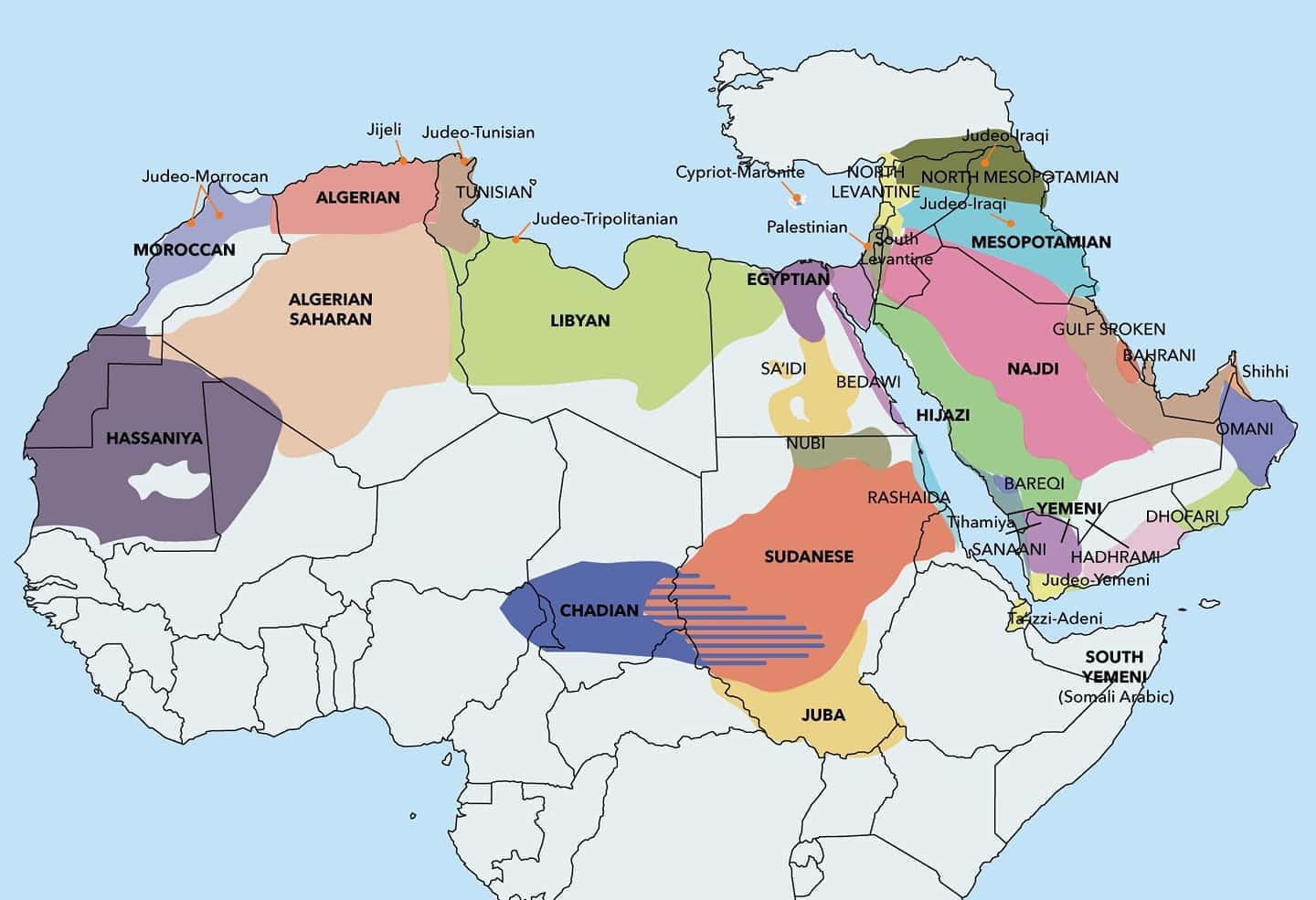
Capturing Linguistic Diversity
There are diverse Arabic dialects, such as Egyptian, Levantine, and Gulf Arabic, each with its own variations and nuances. Generic multilingual models often struggle with this diversity, but Arabic-specific LLMs can better capture these variations, providing more accurate and meaningful responses.
Cultural Alignment
Arabic LLMs can be tailored to understand and reflect cultural and social norms, enabling more respectful and sensitive interactions that resonate with Arabic-speaking users.
Improving AI Performance on Arabic Text
Fine-tuning LLMs specifically for Arabic enhances their ability to understand complex grammatical structures, such as root-based morphology, and handle right-to-left text more effectively. This leads to improvements in NLP tasks such as translation, sentiment analysis, and question-answering.
Fostering Innovation in the MENA Region
The Middle East and North Africa (MENA) region is increasingly investing in AI and digital transformation. Arabic-specific LLMs can empower businesses, governments, and educational institutions within the region to innovate and improve their services.
Bridging Resource Gaps
Arabic, while widely spoken, is considered a low-resource language in terms of AI and NLP resources. By focusing on Arabic, we're helping to bridge this resource gap – bringing more linguistic data, tools, and research attention to an underrepresented language.
Cross-Border Collaboration
Supporting Arabic in LLMs can improve communication and collaboration across the Arabic-speaking world, fostering regional unity and enabling easier access to global markets.
Promoting Localized AI Applications
Arabic LLMs can be tailored to local needs, providing applications such as chatbots, virtual assistants, and content creation tools that are linguistically-aligned and culturally-specific. These applications can enhance user experience in fields such as customer support, education, and social media – delivering value to Arabic-speaking users by meeting their specific needs.
Advancing Research and Talent Development in the Region
Developing Arabic-specific LLMs encourages research in computational linguistics, NLP, and machine learning within Arabic-speaking countries. This not only cultivates local talent and contributes to the region’s AI ecosystem but also brings diverse perspectives to global AI research, enriching the field.
Enabling Accurate Information Access and Knowledge Sharing
Accurate Arabic language processing allows for better dissemination of knowledge, ensuring that Arabic speakers have equitable access to the vast resources available online.
- Nelson Mandela
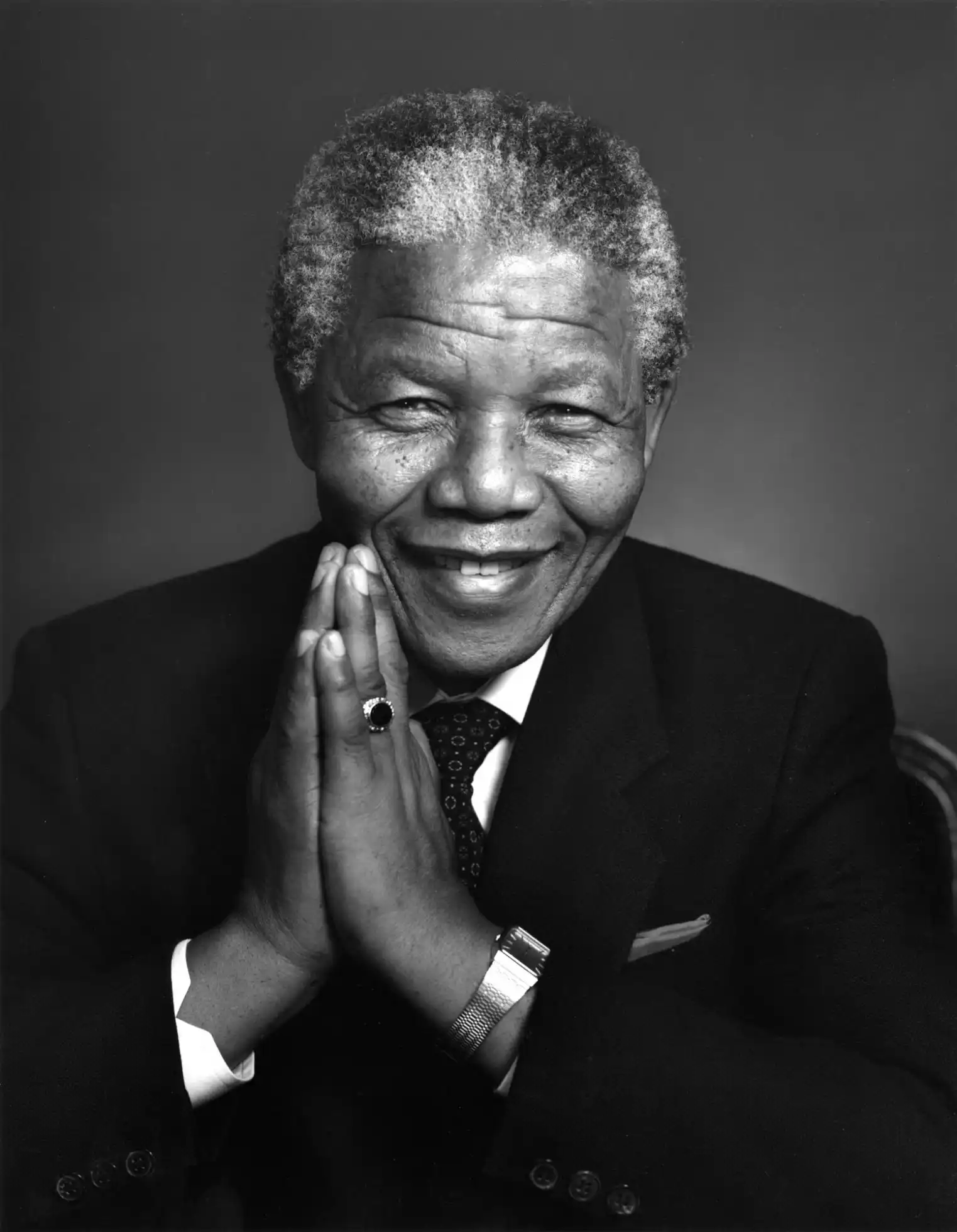
Acknowledgments
We're grateful to the open-source AI community for their continuous contributions and to the Qwen team for their foundational efforts on the Qwen2.5 model series.
Looking Ahead
Arcee AI aims to build accurate and culturally sensitive AI models. In the case of the Arabic language, we strove to linguistic richness, dialectal diversity, and cultural nuances. Our team seeks community collaboration and feedback to refine our Arabic language models and expand domain-specific adaptations. Contributions are key to developing effective future solutions and advancing Arabic NLP, ensuring its significant presence in the multilingual AI landscape.
Learn More About Arcee AI's Models
Do you have a business use case for an LLM or SLM in a non-English language? If so, get in touch and we can tell you about how the Meraj series and our other non-English models are earning immediate ROI for our customers. You can schedule a meeting here.